The Critical Role of Data Labeling in Business Success
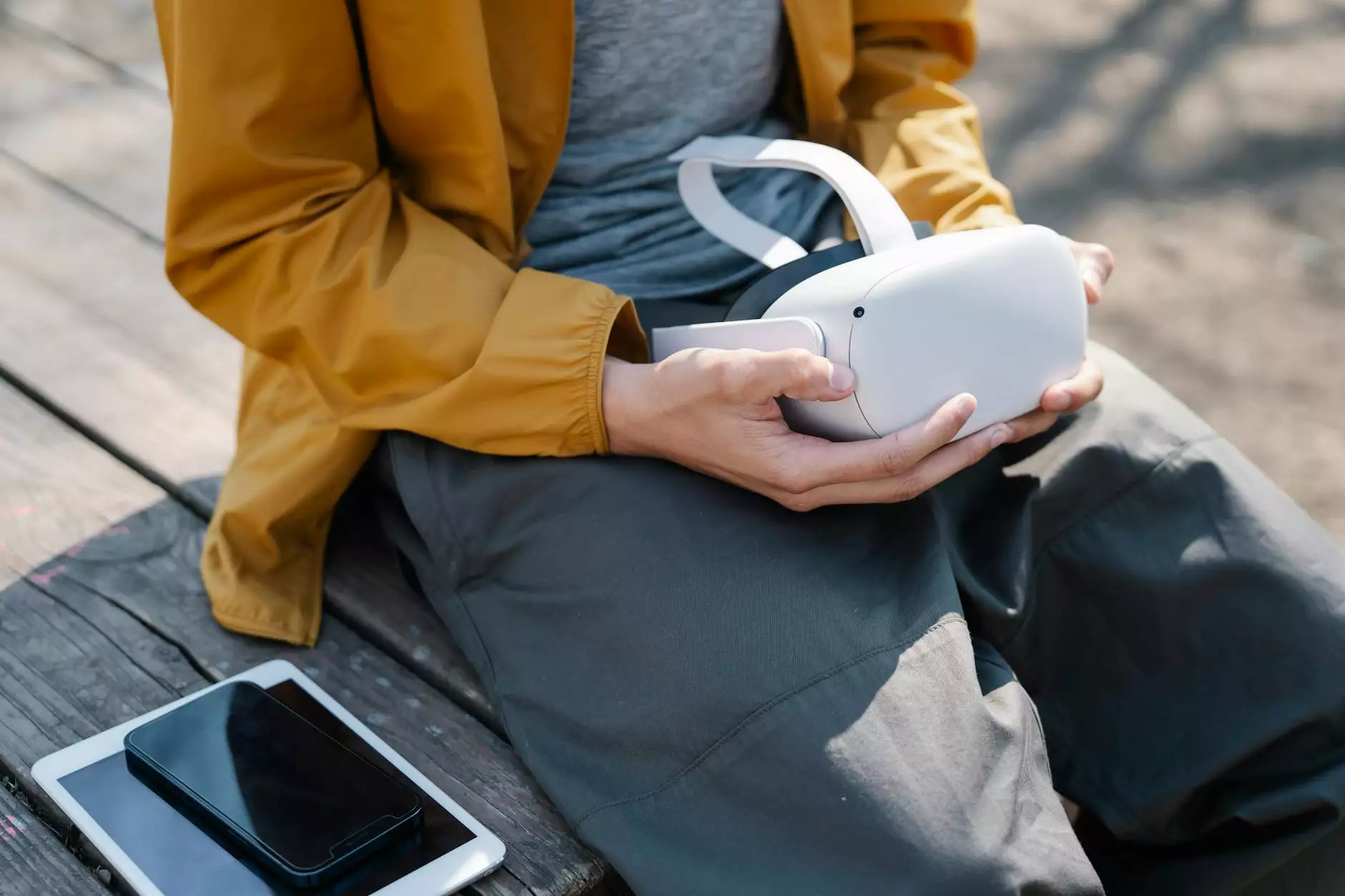
In today's fast-paced and technology-driven environment, the significance of data labeling cannot be overstated. It is a foundational element that propels businesses forward, unlocking transformative potential, particularly in sectors like home services, keys, and locksmiths.
Understanding Data Labeling
Data labeling refers to the process of annotating data so that machine learning algorithms can interpret it correctly. In more practical terms, this means equipping a dataset with meaningful information, facilitating the development of intelligent systems capable of making decisions, predictions, and analyses based on that data.
Why is Data Labeling Essential for Businesses?
Businesses across various industries utilize data labeling for a multitude of reasons:
- Enhanced Accuracy: Properly labeled data allows for more accurate machine learning models, which leads to better predictions and insights.
- Improved Customer Experience: Accurate models help businesses tailor their services to customer preferences, enhancing satisfaction.
- Operational Efficiency: Automating processes through data-driven insights enables businesses to operate more efficiently, reducing costs and time.
- Competitive Advantage: Companies leveraging high-quality labeled data can outperform competitors by offering superior services and smarter solutions.
Applications of Data Labeling in Home Services
The home services industry, which encompasses areas such as plumbing, HVAC, electrical work, and locksmith services, stands to benefit tremendously from data labeling. Here are some applications:
1. Predictive Maintenance
By analyzing labeled data from various home service requests, businesses can predict when maintenance is needed for appliances or systems, thus preventing costly repairs and enhancing customer satisfaction.
2. Customer Service Automation
With well-labeled datasets, companies can train chatbots and virtual assistants that provide quick responses to common customer inquiries, improving efficiency and service quality.
3. Targeted Marketing Campaigns
Data labeling helps businesses understand customer segments better, enabling them to launch targeted marketing campaigns that resonate with specific demographics.
The Process of Data Labeling
The process involved in data labeling is systematic and can be broken down into the following key steps:
- Data Collection: Gather raw data from various sources such as customer inquiries, service requests, and feedback.
- Preprocessing: Clean and organize the data to ensure it is in a usable format for labeling.
- Labeling: Apply labels to the data. This may involve categorizing service types, customer issues, or specific actions taken.
- Verification: Conduct thorough verification processes to ensure that labels are accurate and consistent.
- Integration: Integrate the labeled data into machine learning models and analyze their outputs for further improvements.
Challenges in Data Labeling
While data labeling offers numerous benefits, it also presents challenges that businesses must navigate:
1. Quality Assurance
Maintaining the quality and consistency of labeled data can be arduous, especially when large datasets are involved.
2. Scalability
As businesses grow, the volume of data increases, making it difficult to scale data labeling operations efficiently.
3. Cost Implications
Outsourcing data labeling can incur significant costs, prompting businesses to explore cost-effective in-house solutions.
Outsourcing vs. In-House Data Labeling
Companies often face the decision of whether to outsource their data labeling efforts or to handle them in-house:
- Outsourcing: Engaging third-party services can provide access to specialized skills and technology that may not be available internally, though it typically comes at a higher price.
- In-House: Building an internal team for data labeling can lead to better control over the quality of data but may require significant investment in training and tools.
Best Practices for Effective Data Labeling
To ensure successful data labeling, businesses can adopt the following best practices:
1. Establish Clear Guidelines
Document clear instructions and examples for the data labeling process to ensure that everyone involved understands the requirements.
2. Use Automated Tools
Leverage automation tools to reduce human error and increase efficiency during the labeling process.
3. Regular Training and Feedback
Provide ongoing training for teams involved in data labeling and create feedback loops to continuously improve the process.
4. Monitor Quality Metrics
Regularly assess the quality of labeled data by tracking key performance indicators and making adjustments as necessary.
The Future of Data Labeling in Business
As businesses increasingly rely on machine learning, the demand for effective data labeling is expected to grow exponentially. Innovations in artificial intelligence are making it easier to label data more intelligently and efficiently.
For example, advancements in semi-supervised learning are allowing models to learn from a smaller amount of labeled data, potentially reducing the workload associated with data labeling while maintaining accuracy. As these technologies evolve, businesses must stay ahead of the curve, adopting the latest techniques to maintain their competitive edge.
Conclusion
In summary, data labeling serves as a cornerstone for modern business operations, especially in industries like home services, keys, and locksmiths. By investing in effective data labeling practices, companies can enhance operational efficiency, deliver better customer experiences, and drive meaningful growth. The challenges are many, but the rewards of mastering data labeling are clear. Embracing this critical process will not only prepare businesses for the future but also help them thrive in an ever-evolving marketplace.